Junhao Liang - Tabular Image: A Method to Convert Tabular Data to Images for Convolutional Neural Networks
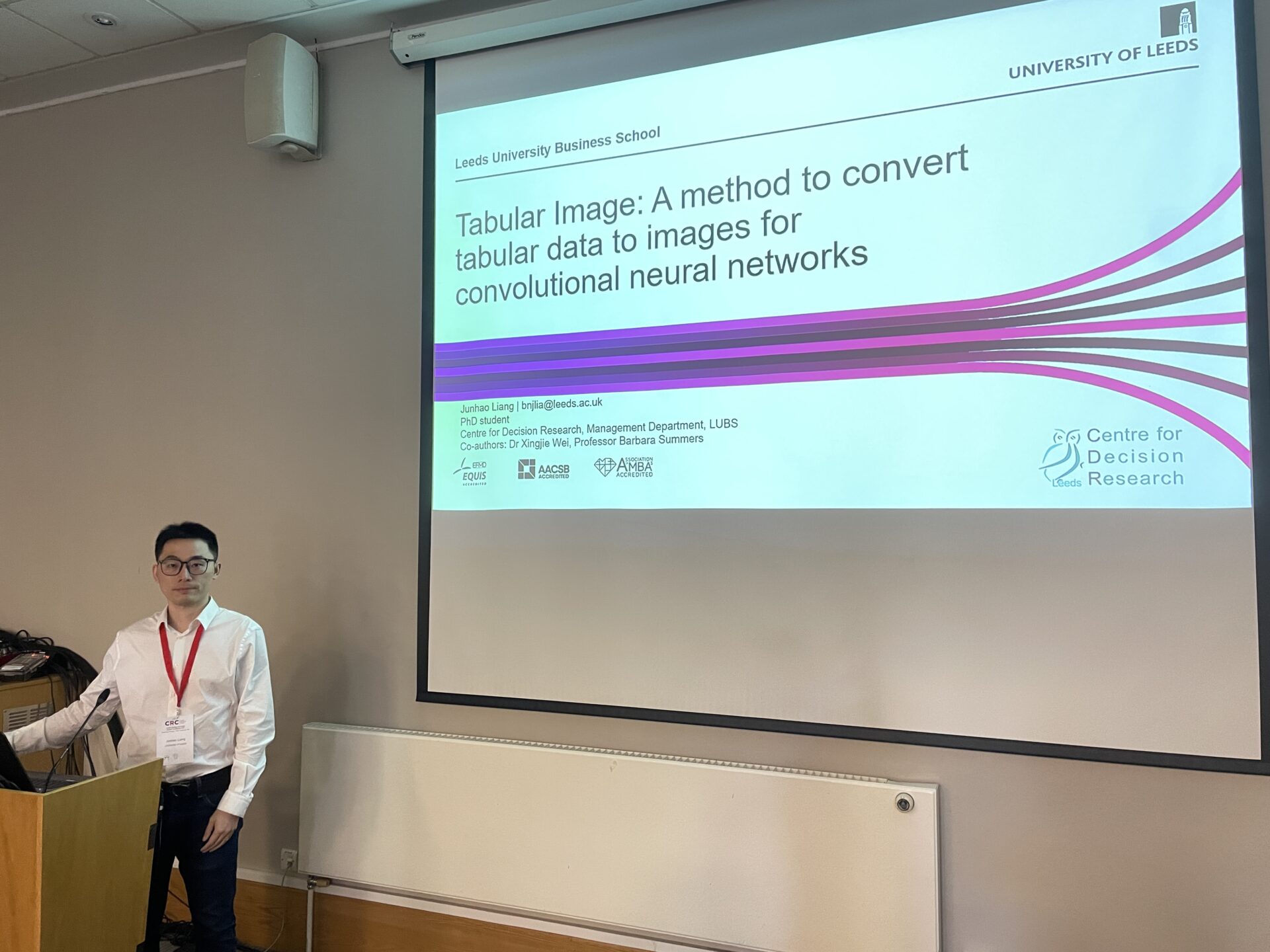
Junhao Liang, a PhD student at the Centre for Decision Research, made a presentation at the 18th meeting of the Credit Scoring and Credit Control conference at the University of Edinburgh held from August 30 to September 1, 2023. Junhao’s presentation, titled "Tabular Image: A Method to Convert Tabular Data to Images for Convolutional Neural Networks”, proposed a novel data transformation method to leverage the powerful two-dimensional convolutional neural networks and big data.
Junhao's presentation sparked a lively discussion among the attendees. His research has the potential to change the way we process and analyse tabular data and could have implications in fields such as credit scoring and fraud detection. The abstract of his presentation is given below.
Tabular Image: A Method to Convert Tabular Data to Images for Convolutional Neural Networks
Improving the forecasting capability of credit scoring models has long been a concern of financial institutions. Because better performances are shown in different domains, various neural networks have been tested to improve loan default prediction on tabular data. However, it is still challenging for neural networks in credit scoring to be applied to credit tabular data and achieve better prediction performance than traditional machine learning models. To overcome the challenge, we propose a novel data transformation method called Tabular Image that converts tabular data into images to take advantage of the powerful two-dimensional convolutional neural networks that perform extremely well on images while mitigating the challenges tabular data poses to deep networks (i.e. a mix of feature types and data sparsity). The Tabular Image can convert tabular data into compact and resilient images compared with existing transformation methods by creatively embedding two crucial measures in credit scoring, the weight of evidence and information value, in the image. Applications to two credit scoring benchmark datasets suggest that a simple two-dimensional convolutional neural network with tabular images can provide state-of-the-art predictive performance. In addition, it is found that our proposed method’s prediction advantage is more evident in the large dataset. The present study raises the possibility of applying two-dimensional convolutional neural networks in credit scoring using a proper data representation method to leverage the powerful two-dimensional convolutional neural networks and big data. Furthermore, our proposed method provides a flexible framework that can be adjusted to suit various tabular datasets in other domains.